Vincent's theorem: Difference between revisions
en>Jonkerz removed meta project template (see Template:Maths banner) |
en>BG19bot m WP:CHECKWIKI error fix for #61. Punctuation goes before References. Do general fixes if a problem exists. - using AWB (9549) |
||
Line 1: | Line 1: | ||
{{Orphan|date=February 2013}} | |||
[[Bayesian inference]] is a statistical tool that can be applied to [[motor learning]], specifically to adaptation. [[neural adaptation|Adaptation]] is a short-term learning process involving gradual improvement in performance in response to a change in sensory information. Bayesian inference is used to describe the way the nervous system combines this sensory information with prior knowledge to estimate the position or other characteristics of something in the environment. Bayesian inference can also be used to show how information from multiple senses (e.g. visual and [[proprioception]]) can be combine for the same purpose. In either case, Bayesian inference dictates that the estimate is most influenced by whichever information is most certain. | |||
==Example: Integrating Prior Knowledge with Sensory Information in Tennis== | |||
[[File:Bayesian tennis court.png|thumb|right|Using Bayesian inference to combine prior and sensory information to estimate the position of a tennis ball]] | |||
A person uses Bayesian inference to create an estimate that is a weighted combination of his current sensory information and his previous knowledge, or prior. This can be illustrated by decisions made in a tennis match.<ref name="Kording06">Körding, K. P., & Wolpert, Daniel M. (2006). Bayesian decision theory in sensorimotor control. Trends in Cognitive Sciences (Vol. 10, pp. 319–326).</ref> If someone plays against a familiar opponent who likes to serve such that the ball strikes on the sideline, one's prior would lead one to place the racket above the sideline to return the serve. However, when one sees the ball moving, it may appear that it will land closer to the middle of the court. Rather than completely following this sensory information or completely following the prior, one would move the racket to a location between the sideline (suggested by the prior) and the point where her eyes indicate the ball will land. | |||
Another key part of Bayesian inference is that the estimate will be closer to the physical state suggested by sensory information if the senses are more accurate and will be closer to the state of the prior if the sensory information is more uncertain than the prior. Extending this to the tennis example, a player facing an opponent for the first time would have little certainty in his/her previous knowledge of the opponent and would therefore have an estimate weighted more heavily on visual information concerning ball position. Alternatively, if one were familiar with one's opponent but were playing in foggy or dark conditions that would hamper sight, sensory information would be less certain and one's estimate would rely more heavily on previous knowledge. | |||
==Statistical Overview== | |||
[[Bayes' Theorem]] states | |||
:<math>P(A|B) = \frac{P(B | A)\, P(A)}{P(B)}. \,</math> | |||
In the language of Bayesian statistics, <math>P(A|B)</math>, or probability of A given B, is called the posterior, while <math>P(B|A)</math> and <math>P(A)</math> are the likelihood and the prior probabilities, respectively.<ref name="Lee">Lee, PM. (2004). Bayesian Statistics: An Introduction. Oxford University Press: London.</ref> <math>P(B)</math> is a constant scaling factor which allows the posterior to be between zero and one. Translating this into the language of motor learning, the prior represents '''previous knowledge''' about the physical state of the thing being observed, the likelihood is '''sensory information''' used to update the prior, and the posterior is the nervous system's '''estimate''' of the physical state. Therefore, for adaptation, Bayes' Theorem can be expressed as | |||
<big>'''estimate''' = '''(previous knowledge''' × '''sensory information)'''/'''scaling factor''' </big> | |||
The 3 terms in the equation above are all probability distributions. To find the estimate in non-probabilistic terms, a weighted sum can be used. | |||
:<math>E = \frac{W_p S + W_s P}{W_p + W_s}. | |||
</math> | |||
where <math>E</math> is the estimate, <math>S</math> is sensory information, <math>P</math> is previous knowledge, and the weighting factors <math>Wp</math> and <math>Ws</math> are the variances of <math>S</math> and <math>P</math>, respectively. Variance is a measure of uncertainty in a variable, so the above equation indicates that higher uncertainty in sensory information causes previous knowledge to have more influence on the estimate and vice versa. | |||
More rigorous mathematical Bayesian descriptions are available [[Bayesian inference|here]] and [[Bayes' Theorem|here]]. | |||
==Reaching== | |||
Many motor tasks exhibit [[neural adaptation|adaptation]] to new sensory information. Bayesian inference has been most commonly studied in reaching. | |||
===Integrating a Prior with Current Sensory Information=== | |||
[[File:One exact data point (A), versus data drawn randomly from a normal distribution (B).jpg|thumb|right|(A) The cursor is represented by one dot with an exact location. (B) The location of the cursor is less certain, because it is somewhere within the cloud of dots]] | |||
Adaptation studies often involve a person reaching for a target without seeing either the target or his/her hand. Instead, the hand is represented by a cursor on a computer screen, which they must move over the target on the screen. In some cases, the cursor is shifted a small distance away from the actual hand position to test how the person responds to changes in visual feedback.<ref name="Kording04">Körding, K. P., & Wolpert, Daniel M. (2004). Bayesian integration in sensorimotor learning. Nature 427:244-7.</ref><ref name="Wei09">Wei, K., Kording, K. (2009). Relevance of error: what drives motor adaptation? J Neurophysiol 101:655-64.</ref> A person learns to counteract this shifts by moving his/her hand an equal and opposite distance from the shift and still moves the cursor to the target, meaning he has developed a prior for this specific shift. When the cursor is then shifted a new, different distance from the same person's reaching hand, the person reaction is consistent with Bayesian inference; the hand moves a distance that is between the old shift (prior) and the new shift (sensory information).<ref name=Kording04/> | |||
If, for the new shift, the cursor is a large cloud of dots instead of one dot (as shown in the figure), the person's sensory information is less clear and will have less influence on how he reacts than the prior will. This supports the Bayesian idea that sensory information with more certainty will have greater influence on a person's adaptation to shifted sensory feedback. | |||
This form of adaptation holds true only when the shift is small compared to the distance the person has to reach to hit the target. A person reaching for a target 15 cm away would adapt to a 2 cm shift of the cursor in a Bayesian way.<ref name=Wei09/> However, if the target were only 5 cm away, a 2 cm shift cursor position (visual information) would be recognized and the person would realize the visual information does not accurately show hand position. Instead, the person would rely on proprioception and prior knowledge to move the hand to the target. | |||
Humans also adapt to changing forces when reaching.<ref name="Force">Kording, K.P., Ku, S., Wolpert, D.M. (2004). Bayesian Integration in force estimation. J Neurophysiol 92:3161-5.</ref> When a force field a person reaches through changes slightly, he modifies his force to maintain reaching in a straight line based partly on a prior force which had been applied earlier. He relies more on the prior if the prior shift is less variable (more certain). | |||
===Integrating Information from Multiple Senses=== | |||
Bayesian inference can also be applied to the way humans combine information about changes in their environment from multiple senses without any consideration of prior knowledge. The two senses that have the strongest influence on how humans' reaching adaptations are vision and proprioception. Typically, proprioception has more weight than vision for adapting hand position in depth - the direction moving toward or away from the person reaching - and vision has more weight in the vertical and horizontal directions.<ref name="VanBeers02">Van Beers, R.J., Wolpert, D.M., Haggard, P. (2002). When feeling is more important than seeing in sensorimotor adaptation. Current Biology 12:834-7.</ref> However, changing conditions can alter the relative influence of these two senses. For example, the influence of vision on adapting hand depth is increased when the hand is passive, while proprioception has more influence when the hand is moving.<ref name=VanBeers02/> Moreover, when vision is reduced (e.g. in darkness) proprioception has more influence on determining hand position.<ref name="Plooy">Plooy, A., Tresilian, J.R., Mon-Williams, M., Wann, J.P. (1998). The contribution of vision and proprioception to judgements of finger proximity. Experimental brain research 118(3):415-20.</ref> This result is consistent with Bayesian inference; when one sense becomes more uncertain, humans increase their reliance on another sense. | |||
==Posture== | |||
Additionally, Bayesian inference has been found to play a part in adaptation of postural control. In one study, for example, subjects use a Wii Balance Board to do a surfing task in which they must move a cursor representing their [[center of pressure (terrestrial locomotion)|center of pressure]] (COP) on a screen.<ref name="Stevenson">Stevenson, I.H., Fernades, H.L., Vilares, I., Wei, K., Kording, K.P. (2009). Bayesian integration and non-linear feedback control in a full-body motor task. PLoS Comp Biol 5(12):1-9.</ref> The Wii surfer got visual information about his/her COP from clouds of dots similar to the one shown in the reaching section. With larger clouds, the surfers were more uncertain and less able to move the COP to the target on the screen. While this result is consistent with Bayesian inference, Bayesian mathematical models did not provide the best predictions of COP motion, perhaps because moving the COP accurately is more mechanically difficult than reaching. Therefore, the extent to which postural movement can be described by Bayesian inference is not yet clear. | |||
==Gait== | |||
Adaptation to shifted feedback also occurs during walking and running. People walking with each foot on a different treadmill belt can adapt their step length when one belt begins moving faster than the other.<ref name="Vasudevan">Vasudevan, E.V., Bastian, A.J. (2010). Split-belt treadmill adaptation shows different functional networks for fast and slow human walking. J Neurophysiol 103:183-191.</ref> Additionally, runners are able to alter their maximum [[ground reaction force]] and leg acceleration when they see a graph of peak leg acceleration.<ref name="Crowell">Crowell, H.P., Milner, C.E., Hamill, J., Davis, I.S. (2010). J Orthopaedic & Sports Physical Therapy 40(4):206-13.</ref> However, to date, no studies have determined if humans adapt their gates using Bayesian inference or not. | |||
==Possible Contradictions to Bayesian Inference== | |||
Some adaptation studies do not support the application of Bayesian inference to motor learning. One study of reaching in a force field found that, rather than being influenced by a prior developed over hundreds of previous reaches, adaptation to subsequent reaches is only influenced by recent memories.<ref name="Scheidt">Scheidt, R.A., Dingwell, J.B., Mussa-Ivaldi, F.A. (2001). Learning to move amid uncertainty. J Neurophysiol 86:971-85.</ref> People reaching in the force field adapted to shifts in the amount of force exerted on the arm, but this adaptation was only affected by the shift in force of the immediately previous reach, not by a well-developed prior knowledge of shifts that had occurred throughout the previous trials of the experiment. This appears to conflict with the application of Bayesian inference to adaptation, but Bayesian adaptation proponents have argued that this particular study required each participant to do only 600 reaches, which is not enough to develop a prior.<ref name=Force/> In reaching studies that show evidence of Bayesian inference, participants typically perform 900 reaches or more.<ref name=Kording04/><ref name=Wei09/> This indicates that, while Bayesian inference is used in adaptation, it is limited in that much previous experience is necessary to develop an influential prior. | |||
==See also== | |||
*[[Bayesian approaches to brain function]] | |||
*[[Neural adaptation]] | |||
*[[Bayesian Inference]] | |||
*[[Perception]] | |||
*[[Motor learning]] | |||
==References== | |||
{{reflist}} | |||
<!--- After listing your sources please cite them using inline citations and place them after the information they cite. Please see http://en.wikipedia.org/wiki/Wikipedia:REFB for instructions on how to add citations. ---> | |||
* | |||
* | |||
* | |||
* | |||
==External links== | |||
*[http://learning.eng.cam.ac.uk/Public/WebHome The Computational and Biological Learning Lab at Cambridge] | |||
*[http://klab.smpp.northwestern.edu Konrad Kording's lab web site] | |||
*[http://www.ap.gatech.edu/Chang/Lab/CNL/APPH6232.html Research for this Wikipedia entry was conducted as a part of a Locomotion Neuromechanics course (APPH 6232) in the School of Applied Physiology at Georgia Tech ] | |||
[[Category:Motor skills]] |
Revision as of 10:16, 27 October 2013
Bayesian inference is a statistical tool that can be applied to motor learning, specifically to adaptation. Adaptation is a short-term learning process involving gradual improvement in performance in response to a change in sensory information. Bayesian inference is used to describe the way the nervous system combines this sensory information with prior knowledge to estimate the position or other characteristics of something in the environment. Bayesian inference can also be used to show how information from multiple senses (e.g. visual and proprioception) can be combine for the same purpose. In either case, Bayesian inference dictates that the estimate is most influenced by whichever information is most certain.
Example: Integrating Prior Knowledge with Sensory Information in Tennis
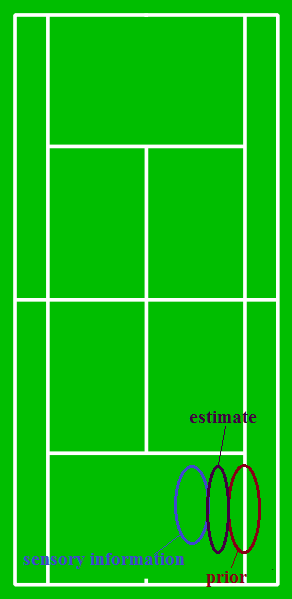
A person uses Bayesian inference to create an estimate that is a weighted combination of his current sensory information and his previous knowledge, or prior. This can be illustrated by decisions made in a tennis match.[1] If someone plays against a familiar opponent who likes to serve such that the ball strikes on the sideline, one's prior would lead one to place the racket above the sideline to return the serve. However, when one sees the ball moving, it may appear that it will land closer to the middle of the court. Rather than completely following this sensory information or completely following the prior, one would move the racket to a location between the sideline (suggested by the prior) and the point where her eyes indicate the ball will land.
Another key part of Bayesian inference is that the estimate will be closer to the physical state suggested by sensory information if the senses are more accurate and will be closer to the state of the prior if the sensory information is more uncertain than the prior. Extending this to the tennis example, a player facing an opponent for the first time would have little certainty in his/her previous knowledge of the opponent and would therefore have an estimate weighted more heavily on visual information concerning ball position. Alternatively, if one were familiar with one's opponent but were playing in foggy or dark conditions that would hamper sight, sensory information would be less certain and one's estimate would rely more heavily on previous knowledge.
Statistical Overview
Bayes' Theorem states
In the language of Bayesian statistics, , or probability of A given B, is called the posterior, while and are the likelihood and the prior probabilities, respectively.[2] is a constant scaling factor which allows the posterior to be between zero and one. Translating this into the language of motor learning, the prior represents previous knowledge about the physical state of the thing being observed, the likelihood is sensory information used to update the prior, and the posterior is the nervous system's estimate of the physical state. Therefore, for adaptation, Bayes' Theorem can be expressed as
estimate = (previous knowledge × sensory information)/scaling factor
The 3 terms in the equation above are all probability distributions. To find the estimate in non-probabilistic terms, a weighted sum can be used.
where is the estimate, is sensory information, is previous knowledge, and the weighting factors and are the variances of and , respectively. Variance is a measure of uncertainty in a variable, so the above equation indicates that higher uncertainty in sensory information causes previous knowledge to have more influence on the estimate and vice versa.
More rigorous mathematical Bayesian descriptions are available here and here.
Reaching
Many motor tasks exhibit adaptation to new sensory information. Bayesian inference has been most commonly studied in reaching.
Integrating a Prior with Current Sensory Information

Adaptation studies often involve a person reaching for a target without seeing either the target or his/her hand. Instead, the hand is represented by a cursor on a computer screen, which they must move over the target on the screen. In some cases, the cursor is shifted a small distance away from the actual hand position to test how the person responds to changes in visual feedback.[3][4] A person learns to counteract this shifts by moving his/her hand an equal and opposite distance from the shift and still moves the cursor to the target, meaning he has developed a prior for this specific shift. When the cursor is then shifted a new, different distance from the same person's reaching hand, the person reaction is consistent with Bayesian inference; the hand moves a distance that is between the old shift (prior) and the new shift (sensory information).[3]
If, for the new shift, the cursor is a large cloud of dots instead of one dot (as shown in the figure), the person's sensory information is less clear and will have less influence on how he reacts than the prior will. This supports the Bayesian idea that sensory information with more certainty will have greater influence on a person's adaptation to shifted sensory feedback.
This form of adaptation holds true only when the shift is small compared to the distance the person has to reach to hit the target. A person reaching for a target 15 cm away would adapt to a 2 cm shift of the cursor in a Bayesian way.[4] However, if the target were only 5 cm away, a 2 cm shift cursor position (visual information) would be recognized and the person would realize the visual information does not accurately show hand position. Instead, the person would rely on proprioception and prior knowledge to move the hand to the target.
Humans also adapt to changing forces when reaching.[5] When a force field a person reaches through changes slightly, he modifies his force to maintain reaching in a straight line based partly on a prior force which had been applied earlier. He relies more on the prior if the prior shift is less variable (more certain).
Integrating Information from Multiple Senses
Bayesian inference can also be applied to the way humans combine information about changes in their environment from multiple senses without any consideration of prior knowledge. The two senses that have the strongest influence on how humans' reaching adaptations are vision and proprioception. Typically, proprioception has more weight than vision for adapting hand position in depth - the direction moving toward or away from the person reaching - and vision has more weight in the vertical and horizontal directions.[6] However, changing conditions can alter the relative influence of these two senses. For example, the influence of vision on adapting hand depth is increased when the hand is passive, while proprioception has more influence when the hand is moving.[6] Moreover, when vision is reduced (e.g. in darkness) proprioception has more influence on determining hand position.[7] This result is consistent with Bayesian inference; when one sense becomes more uncertain, humans increase their reliance on another sense.
Posture
Additionally, Bayesian inference has been found to play a part in adaptation of postural control. In one study, for example, subjects use a Wii Balance Board to do a surfing task in which they must move a cursor representing their center of pressure (COP) on a screen.[8] The Wii surfer got visual information about his/her COP from clouds of dots similar to the one shown in the reaching section. With larger clouds, the surfers were more uncertain and less able to move the COP to the target on the screen. While this result is consistent with Bayesian inference, Bayesian mathematical models did not provide the best predictions of COP motion, perhaps because moving the COP accurately is more mechanically difficult than reaching. Therefore, the extent to which postural movement can be described by Bayesian inference is not yet clear.
Gait
Adaptation to shifted feedback also occurs during walking and running. People walking with each foot on a different treadmill belt can adapt their step length when one belt begins moving faster than the other.[9] Additionally, runners are able to alter their maximum ground reaction force and leg acceleration when they see a graph of peak leg acceleration.[10] However, to date, no studies have determined if humans adapt their gates using Bayesian inference or not.
Possible Contradictions to Bayesian Inference
Some adaptation studies do not support the application of Bayesian inference to motor learning. One study of reaching in a force field found that, rather than being influenced by a prior developed over hundreds of previous reaches, adaptation to subsequent reaches is only influenced by recent memories.[11] People reaching in the force field adapted to shifts in the amount of force exerted on the arm, but this adaptation was only affected by the shift in force of the immediately previous reach, not by a well-developed prior knowledge of shifts that had occurred throughout the previous trials of the experiment. This appears to conflict with the application of Bayesian inference to adaptation, but Bayesian adaptation proponents have argued that this particular study required each participant to do only 600 reaches, which is not enough to develop a prior.[5] In reaching studies that show evidence of Bayesian inference, participants typically perform 900 reaches or more.[3][4] This indicates that, while Bayesian inference is used in adaptation, it is limited in that much previous experience is necessary to develop an influential prior.
See also
- Bayesian approaches to brain function
- Neural adaptation
- Bayesian Inference
- Perception
- Motor learning
References
43 year old Petroleum Engineer Harry from Deep River, usually spends time with hobbies and interests like renting movies, property developers in singapore new condominium and vehicle racing. Constantly enjoys going to destinations like Camino Real de Tierra Adentro.
External links
- The Computational and Biological Learning Lab at Cambridge
- Konrad Kording's lab web site
- Research for this Wikipedia entry was conducted as a part of a Locomotion Neuromechanics course (APPH 6232) in the School of Applied Physiology at Georgia Tech
- ↑ Körding, K. P., & Wolpert, Daniel M. (2006). Bayesian decision theory in sensorimotor control. Trends in Cognitive Sciences (Vol. 10, pp. 319–326).
- ↑ Lee, PM. (2004). Bayesian Statistics: An Introduction. Oxford University Press: London.
- ↑ 3.0 3.1 3.2 Körding, K. P., & Wolpert, Daniel M. (2004). Bayesian integration in sensorimotor learning. Nature 427:244-7.
- ↑ 4.0 4.1 4.2 Wei, K., Kording, K. (2009). Relevance of error: what drives motor adaptation? J Neurophysiol 101:655-64.
- ↑ 5.0 5.1 Kording, K.P., Ku, S., Wolpert, D.M. (2004). Bayesian Integration in force estimation. J Neurophysiol 92:3161-5.
- ↑ 6.0 6.1 Van Beers, R.J., Wolpert, D.M., Haggard, P. (2002). When feeling is more important than seeing in sensorimotor adaptation. Current Biology 12:834-7.
- ↑ Plooy, A., Tresilian, J.R., Mon-Williams, M., Wann, J.P. (1998). The contribution of vision and proprioception to judgements of finger proximity. Experimental brain research 118(3):415-20.
- ↑ Stevenson, I.H., Fernades, H.L., Vilares, I., Wei, K., Kording, K.P. (2009). Bayesian integration and non-linear feedback control in a full-body motor task. PLoS Comp Biol 5(12):1-9.
- ↑ Vasudevan, E.V., Bastian, A.J. (2010). Split-belt treadmill adaptation shows different functional networks for fast and slow human walking. J Neurophysiol 103:183-191.
- ↑ Crowell, H.P., Milner, C.E., Hamill, J., Davis, I.S. (2010). J Orthopaedic & Sports Physical Therapy 40(4):206-13.
- ↑ Scheidt, R.A., Dingwell, J.B., Mussa-Ivaldi, F.A. (2001). Learning to move amid uncertainty. J Neurophysiol 86:971-85.